How to interpret this form of Heaps' Law? Planned maintenance scheduled April 17/18, 2019 at 00:00UTC (8:00pm US/Eastern) Announcing the arrival of Valued Associate #679: Cesar Manara Unicorn Meta Zoo #1: Why another podcast?Are there natural languages that do not obey Zipf's law?What is the relationship between lambda calculus and logical form?Calculating writing system efficiency with respect to reading ambiguity?How can I calculate if the difference between two word frequencies in one corpus is significant?How can I automatically build a domain specific corpus from scratch?How to proceed with this Maasai translation?How to quantify and compare different ways of segmenting and transliterating (reading) a text in terms of uncertainty/leeway?Syntax tree for this sentenceThe determiner “a” in lambda formHow can I know if a log-likelihood score is high enough?
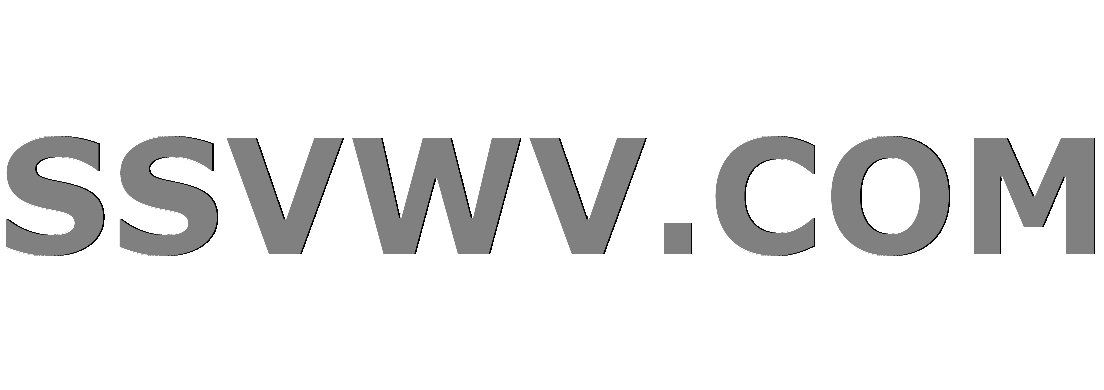
Multi tool use
How to deal with a team lead who never gives me credit?
Withdrew £2800, but only £2000 shows as withdrawn on online banking; what are my obligations?
Why aren't air breathing engines used as small first stages
Check which numbers satisfy the condition [A*B*C = A! + B! + C!]
Can a non-EU citizen traveling with me come with me through the EU passport line?
What's the meaning of 間時肆拾貳 at a car parking sign
Selecting the same column from Different rows Based on Different Criteria
Why didn't this character "real die" when they blew their stack out in Altered Carbon?
How to align text above triangle figure
Is it ethical to give a final exam after the professor has quit before teaching the remaining chapters of the course?
What to do with chalk when deepwater soloing?
How to run gsettings for another user Ubuntu 18.04.2 LTS
Fundamental Solution of the Pell Equation
Do I really need recursive chmod to restrict access to a folder?
What is the meaning of the new sigil in Game of Thrones Season 8 intro?
How would the world control an invulnerable immortal mass murderer?
How to call a function with default parameter through a pointer to function that is the return of another function?
How come Sam didn't become Lord of Horn Hill?
Should I discuss the type of campaign with my players?
Using et al. for a last / senior author rather than for a first author
How does debian/ubuntu knows a package has a updated version
Why did the IBM 650 use bi-quinary?
Single word antonym of "flightless"
Echoing a tail command produces unexpected output?
How to interpret this form of Heaps' Law?
Planned maintenance scheduled April 17/18, 2019 at 00:00UTC (8:00pm US/Eastern)
Announcing the arrival of Valued Associate #679: Cesar Manara
Unicorn Meta Zoo #1: Why another podcast?Are there natural languages that do not obey Zipf's law?What is the relationship between lambda calculus and logical form?Calculating writing system efficiency with respect to reading ambiguity?How can I calculate if the difference between two word frequencies in one corpus is significant?How can I automatically build a domain specific corpus from scratch?How to proceed with this Maasai translation?How to quantify and compare different ways of segmenting and transliterating (reading) a text in terms of uncertainty/leeway?Syntax tree for this sentenceThe determiner “a” in lambda formHow can I know if a log-likelihood score is high enough?
Heaps' Law basically is an empirical function that says the number of distinct words you'll find in a document grows as a function to the length of the document. The equation given in the Wikipedia link is
where $V_R$ is the number of distinct words in a document of size $n$, and $K$ and $beta$ are free parameters that are chosen empirically (usually $0 le K le 100$ and $0.4 le beta le 0.6$).
I'm currently following a course on Youtube called Deep Learning for NLP by Oxford University and DeepMind. There is a slide in a lecture that demonstrates Heaps' Law in a rather different way:
The equation given with the logarithms apparently is also Heaps' Law. The fastest growing curve is a corpus for Twitter data and the slowest is for the Wall Street Journal. Tweets usually have less structure and more spelling errors, etc. compared to the WSJ which would explain the faster-growing curve.
The main question that I had is how Heaps' Law seems to have taken on the form that the author has given? It's a bit of a reach but the author didn't specify what any of these parameters are and I was wondering if anybody might be familiar with Heaps' Law to give me some advise on how to solve my question.
computational-linguistics corpora quantitative-linguistics
add a comment |
Heaps' Law basically is an empirical function that says the number of distinct words you'll find in a document grows as a function to the length of the document. The equation given in the Wikipedia link is
where $V_R$ is the number of distinct words in a document of size $n$, and $K$ and $beta$ are free parameters that are chosen empirically (usually $0 le K le 100$ and $0.4 le beta le 0.6$).
I'm currently following a course on Youtube called Deep Learning for NLP by Oxford University and DeepMind. There is a slide in a lecture that demonstrates Heaps' Law in a rather different way:
The equation given with the logarithms apparently is also Heaps' Law. The fastest growing curve is a corpus for Twitter data and the slowest is for the Wall Street Journal. Tweets usually have less structure and more spelling errors, etc. compared to the WSJ which would explain the faster-growing curve.
The main question that I had is how Heaps' Law seems to have taken on the form that the author has given? It's a bit of a reach but the author didn't specify what any of these parameters are and I was wondering if anybody might be familiar with Heaps' Law to give me some advise on how to solve my question.
computational-linguistics corpora quantitative-linguistics
add a comment |
Heaps' Law basically is an empirical function that says the number of distinct words you'll find in a document grows as a function to the length of the document. The equation given in the Wikipedia link is
where $V_R$ is the number of distinct words in a document of size $n$, and $K$ and $beta$ are free parameters that are chosen empirically (usually $0 le K le 100$ and $0.4 le beta le 0.6$).
I'm currently following a course on Youtube called Deep Learning for NLP by Oxford University and DeepMind. There is a slide in a lecture that demonstrates Heaps' Law in a rather different way:
The equation given with the logarithms apparently is also Heaps' Law. The fastest growing curve is a corpus for Twitter data and the slowest is for the Wall Street Journal. Tweets usually have less structure and more spelling errors, etc. compared to the WSJ which would explain the faster-growing curve.
The main question that I had is how Heaps' Law seems to have taken on the form that the author has given? It's a bit of a reach but the author didn't specify what any of these parameters are and I was wondering if anybody might be familiar with Heaps' Law to give me some advise on how to solve my question.
computational-linguistics corpora quantitative-linguistics
Heaps' Law basically is an empirical function that says the number of distinct words you'll find in a document grows as a function to the length of the document. The equation given in the Wikipedia link is
where $V_R$ is the number of distinct words in a document of size $n$, and $K$ and $beta$ are free parameters that are chosen empirically (usually $0 le K le 100$ and $0.4 le beta le 0.6$).
I'm currently following a course on Youtube called Deep Learning for NLP by Oxford University and DeepMind. There is a slide in a lecture that demonstrates Heaps' Law in a rather different way:
The equation given with the logarithms apparently is also Heaps' Law. The fastest growing curve is a corpus for Twitter data and the slowest is for the Wall Street Journal. Tweets usually have less structure and more spelling errors, etc. compared to the WSJ which would explain the faster-growing curve.
The main question that I had is how Heaps' Law seems to have taken on the form that the author has given? It's a bit of a reach but the author didn't specify what any of these parameters are and I was wondering if anybody might be familiar with Heaps' Law to give me some advise on how to solve my question.
computational-linguistics corpora quantitative-linguistics
computational-linguistics corpora quantitative-linguistics
edited Apr 9 at 15:20
jknappen
11.8k22854
11.8k22854
asked Apr 9 at 14:11
SeankalaSeankala
1235
1235
add a comment |
add a comment |
2 Answers
2
active
oldest
votes
A straightforward rewriting of the Wikipedia formula gives
log V_R(n) = log K*n^beta
= log K + log n^beta
= log K + beta*log n
This allows us to identify K=C
and beta=-alpha
(probably the WSJ uses a different formulation of Heaps' law
V_R (n) = fracKn^alpha
). The remaining b is a strange additional parameter not present in the original formulation of the law (and irrelevant, too, because the law is about large numbers where n-b is approximately equal to n).
Thanks for the answer. I tried to apply logarithms to each side but it didn't come to mind thatK = C
andβ = -α
. This may also sound like a bit of an out-of-placed question, but would you happen to know what a "singleton" in this context is? My knowledge of set theory tells me that it means a single perceptual unit, or a word in this context.
– Seankala
Apr 9 at 15:34
From the small context given, I can only guess what a singleton could be here. My guess is that it refers to a hapax legomenon, i.e., a word form that occurs exactly once in the corpus (or sample).
– jknappen
Apr 9 at 16:07
alpha in the chart is notably not in the same range as implied for beta in the question. I'm not sure whether that makes a huge difference. I guess it does.
– vectory
Apr 9 at 17:59
singleton 34.3%, 70% must mean hapax legomenon percentage of new words. However, that still seems quite high. Edit: that wouldn't even make sense, if every word is new at some point, unless they don't count a seizable number of basic vocabulary as new, like, compact OED sized.
– vectory
Apr 9 at 18:02
add a comment |
I am going to guess and I hope someone has a clearer idea.
The question is interesting from a (my) novice math perspective, the wording suggests it was moved from mathoverflow.se?
From a basic linguistic perspective, there is little to no difference, all you need is a slowly decreasing slope. Both describe standard distributions, a concepts that's naturally observed in nature, from the distribution of raindrops to the dispersion of a laser beam. The specific choice depends on an accurate model. If it's instead just chosen to fit the data somehow, it doesn't hold much explanatory power, but it's a heuristic. For the specifics you should check out datascience.se, or whatever it's called where statistics are treated (compression of text is also rather important in signal processing).
The first one, V = k * n ^ b, is akin to the area of a circle, A = pi * r ^ 2, but inverted (taking the square root) and with a random factor, instead of pi, which can be pictured various ways, e.g. as a circle projected onto a wavy area or through a lense (doesn't really matter unless there's a specific need). If b is not exactly 0.5 the picture is a little different, but not really. The point is, this appears as the inverse square law, e.g. if a light cone hits a wall further away, the radius will increase linearly, but the power per square are will diminish proportionally with the inverse square of the distance. A^1/2 ~ r. The length of a text, n, increases likewise proportionally with the number of new words, n^0.5 ~ V. In other words, the text grows squarely with each new new word. That's also proportional to the circumference.
The second one seems more elaborate. I too have no idea what the extra variables are. Removing the logarithm we have *f(w) = C * (r(w)-b)^(-alpha)*. And transposed 1/C * (r(w)-b)^a = 1 / f(w). This is in principle the same polynomial form as V=K*n^b with several new parameters, if it were that V = 1 / f(w), k = 1/C, n = (r(w) - b), beta = -alpha.
There are a few notable differences. What's with those parameters? I'd assume the following:
b is likely a threshold under which the distribution is useless, because if r(w)Basic vocabulary.
If C is a constant as usual, then writing log(C), which would be constant as well, might just be a courtesy to ease solving for (w). It's inversely proportional to k, but that shouldn't trouble us now. One way or another it will be normalizing the results. I'm keen to assume that it means Corpus, but that gives me troubles. [todo]
That leaves alpha to be explained, which seems to be a variable nudge factor determined per corpus by a specific statistical procedure for error correction.
The last one is crucial. Raising to a negative power of alpha (=reciproke of the power of alpha) is not quite the same as taking the square root (power of 0.5). But it is similar in effect because the ranges of the exponents are also different, b < 1 < alpha. The very important difference is that the number of new words will tend to zero as the number of typed words tends to infinity--which, if I may say so, is a rather real possibility with twitter ;-) While the old formula would require ever new words to grow the text.
Somehow I'm trying to see 1/f as a derivative, compared to mechanical accelleration. But I'll rather leave the rest of the exercise to the reader. Please add a link to the video to your question. thx bye
add a comment |
Your Answer
StackExchange.ready(function()
var channelOptions =
tags: "".split(" "),
id: "312"
;
initTagRenderer("".split(" "), "".split(" "), channelOptions);
StackExchange.using("externalEditor", function()
// Have to fire editor after snippets, if snippets enabled
if (StackExchange.settings.snippets.snippetsEnabled)
StackExchange.using("snippets", function()
createEditor();
);
else
createEditor();
);
function createEditor()
StackExchange.prepareEditor(
heartbeatType: 'answer',
autoActivateHeartbeat: false,
convertImagesToLinks: false,
noModals: true,
showLowRepImageUploadWarning: true,
reputationToPostImages: null,
bindNavPrevention: true,
postfix: "",
imageUploader:
brandingHtml: "Powered by u003ca class="icon-imgur-white" href="https://imgur.com/"u003eu003c/au003e",
contentPolicyHtml: "User contributions licensed under u003ca href="https://creativecommons.org/licenses/by-sa/3.0/"u003ecc by-sa 3.0 with attribution requiredu003c/au003e u003ca href="https://stackoverflow.com/legal/content-policy"u003e(content policy)u003c/au003e",
allowUrls: true
,
noCode: true, onDemand: true,
discardSelector: ".discard-answer"
,immediatelyShowMarkdownHelp:true
);
);
Sign up or log in
StackExchange.ready(function ()
StackExchange.helpers.onClickDraftSave('#login-link');
);
Sign up using Google
Sign up using Facebook
Sign up using Email and Password
Post as a guest
Required, but never shown
StackExchange.ready(
function ()
StackExchange.openid.initPostLogin('.new-post-login', 'https%3a%2f%2flinguistics.stackexchange.com%2fquestions%2f31118%2fhow-to-interpret-this-form-of-heaps-law%23new-answer', 'question_page');
);
Post as a guest
Required, but never shown
2 Answers
2
active
oldest
votes
2 Answers
2
active
oldest
votes
active
oldest
votes
active
oldest
votes
A straightforward rewriting of the Wikipedia formula gives
log V_R(n) = log K*n^beta
= log K + log n^beta
= log K + beta*log n
This allows us to identify K=C
and beta=-alpha
(probably the WSJ uses a different formulation of Heaps' law
V_R (n) = fracKn^alpha
). The remaining b is a strange additional parameter not present in the original formulation of the law (and irrelevant, too, because the law is about large numbers where n-b is approximately equal to n).
Thanks for the answer. I tried to apply logarithms to each side but it didn't come to mind thatK = C
andβ = -α
. This may also sound like a bit of an out-of-placed question, but would you happen to know what a "singleton" in this context is? My knowledge of set theory tells me that it means a single perceptual unit, or a word in this context.
– Seankala
Apr 9 at 15:34
From the small context given, I can only guess what a singleton could be here. My guess is that it refers to a hapax legomenon, i.e., a word form that occurs exactly once in the corpus (or sample).
– jknappen
Apr 9 at 16:07
alpha in the chart is notably not in the same range as implied for beta in the question. I'm not sure whether that makes a huge difference. I guess it does.
– vectory
Apr 9 at 17:59
singleton 34.3%, 70% must mean hapax legomenon percentage of new words. However, that still seems quite high. Edit: that wouldn't even make sense, if every word is new at some point, unless they don't count a seizable number of basic vocabulary as new, like, compact OED sized.
– vectory
Apr 9 at 18:02
add a comment |
A straightforward rewriting of the Wikipedia formula gives
log V_R(n) = log K*n^beta
= log K + log n^beta
= log K + beta*log n
This allows us to identify K=C
and beta=-alpha
(probably the WSJ uses a different formulation of Heaps' law
V_R (n) = fracKn^alpha
). The remaining b is a strange additional parameter not present in the original formulation of the law (and irrelevant, too, because the law is about large numbers where n-b is approximately equal to n).
Thanks for the answer. I tried to apply logarithms to each side but it didn't come to mind thatK = C
andβ = -α
. This may also sound like a bit of an out-of-placed question, but would you happen to know what a "singleton" in this context is? My knowledge of set theory tells me that it means a single perceptual unit, or a word in this context.
– Seankala
Apr 9 at 15:34
From the small context given, I can only guess what a singleton could be here. My guess is that it refers to a hapax legomenon, i.e., a word form that occurs exactly once in the corpus (or sample).
– jknappen
Apr 9 at 16:07
alpha in the chart is notably not in the same range as implied for beta in the question. I'm not sure whether that makes a huge difference. I guess it does.
– vectory
Apr 9 at 17:59
singleton 34.3%, 70% must mean hapax legomenon percentage of new words. However, that still seems quite high. Edit: that wouldn't even make sense, if every word is new at some point, unless they don't count a seizable number of basic vocabulary as new, like, compact OED sized.
– vectory
Apr 9 at 18:02
add a comment |
A straightforward rewriting of the Wikipedia formula gives
log V_R(n) = log K*n^beta
= log K + log n^beta
= log K + beta*log n
This allows us to identify K=C
and beta=-alpha
(probably the WSJ uses a different formulation of Heaps' law
V_R (n) = fracKn^alpha
). The remaining b is a strange additional parameter not present in the original formulation of the law (and irrelevant, too, because the law is about large numbers where n-b is approximately equal to n).
A straightforward rewriting of the Wikipedia formula gives
log V_R(n) = log K*n^beta
= log K + log n^beta
= log K + beta*log n
This allows us to identify K=C
and beta=-alpha
(probably the WSJ uses a different formulation of Heaps' law
V_R (n) = fracKn^alpha
). The remaining b is a strange additional parameter not present in the original formulation of the law (and irrelevant, too, because the law is about large numbers where n-b is approximately equal to n).
edited Apr 9 at 15:27
answered Apr 9 at 15:16
jknappenjknappen
11.8k22854
11.8k22854
Thanks for the answer. I tried to apply logarithms to each side but it didn't come to mind thatK = C
andβ = -α
. This may also sound like a bit of an out-of-placed question, but would you happen to know what a "singleton" in this context is? My knowledge of set theory tells me that it means a single perceptual unit, or a word in this context.
– Seankala
Apr 9 at 15:34
From the small context given, I can only guess what a singleton could be here. My guess is that it refers to a hapax legomenon, i.e., a word form that occurs exactly once in the corpus (or sample).
– jknappen
Apr 9 at 16:07
alpha in the chart is notably not in the same range as implied for beta in the question. I'm not sure whether that makes a huge difference. I guess it does.
– vectory
Apr 9 at 17:59
singleton 34.3%, 70% must mean hapax legomenon percentage of new words. However, that still seems quite high. Edit: that wouldn't even make sense, if every word is new at some point, unless they don't count a seizable number of basic vocabulary as new, like, compact OED sized.
– vectory
Apr 9 at 18:02
add a comment |
Thanks for the answer. I tried to apply logarithms to each side but it didn't come to mind thatK = C
andβ = -α
. This may also sound like a bit of an out-of-placed question, but would you happen to know what a "singleton" in this context is? My knowledge of set theory tells me that it means a single perceptual unit, or a word in this context.
– Seankala
Apr 9 at 15:34
From the small context given, I can only guess what a singleton could be here. My guess is that it refers to a hapax legomenon, i.e., a word form that occurs exactly once in the corpus (or sample).
– jknappen
Apr 9 at 16:07
alpha in the chart is notably not in the same range as implied for beta in the question. I'm not sure whether that makes a huge difference. I guess it does.
– vectory
Apr 9 at 17:59
singleton 34.3%, 70% must mean hapax legomenon percentage of new words. However, that still seems quite high. Edit: that wouldn't even make sense, if every word is new at some point, unless they don't count a seizable number of basic vocabulary as new, like, compact OED sized.
– vectory
Apr 9 at 18:02
Thanks for the answer. I tried to apply logarithms to each side but it didn't come to mind that
K = C
and β = -α
. This may also sound like a bit of an out-of-placed question, but would you happen to know what a "singleton" in this context is? My knowledge of set theory tells me that it means a single perceptual unit, or a word in this context.– Seankala
Apr 9 at 15:34
Thanks for the answer. I tried to apply logarithms to each side but it didn't come to mind that
K = C
and β = -α
. This may also sound like a bit of an out-of-placed question, but would you happen to know what a "singleton" in this context is? My knowledge of set theory tells me that it means a single perceptual unit, or a word in this context.– Seankala
Apr 9 at 15:34
From the small context given, I can only guess what a singleton could be here. My guess is that it refers to a hapax legomenon, i.e., a word form that occurs exactly once in the corpus (or sample).
– jknappen
Apr 9 at 16:07
From the small context given, I can only guess what a singleton could be here. My guess is that it refers to a hapax legomenon, i.e., a word form that occurs exactly once in the corpus (or sample).
– jknappen
Apr 9 at 16:07
alpha in the chart is notably not in the same range as implied for beta in the question. I'm not sure whether that makes a huge difference. I guess it does.
– vectory
Apr 9 at 17:59
alpha in the chart is notably not in the same range as implied for beta in the question. I'm not sure whether that makes a huge difference. I guess it does.
– vectory
Apr 9 at 17:59
singleton 34.3%, 70% must mean hapax legomenon percentage of new words. However, that still seems quite high. Edit: that wouldn't even make sense, if every word is new at some point, unless they don't count a seizable number of basic vocabulary as new, like, compact OED sized.
– vectory
Apr 9 at 18:02
singleton 34.3%, 70% must mean hapax legomenon percentage of new words. However, that still seems quite high. Edit: that wouldn't even make sense, if every word is new at some point, unless they don't count a seizable number of basic vocabulary as new, like, compact OED sized.
– vectory
Apr 9 at 18:02
add a comment |
I am going to guess and I hope someone has a clearer idea.
The question is interesting from a (my) novice math perspective, the wording suggests it was moved from mathoverflow.se?
From a basic linguistic perspective, there is little to no difference, all you need is a slowly decreasing slope. Both describe standard distributions, a concepts that's naturally observed in nature, from the distribution of raindrops to the dispersion of a laser beam. The specific choice depends on an accurate model. If it's instead just chosen to fit the data somehow, it doesn't hold much explanatory power, but it's a heuristic. For the specifics you should check out datascience.se, or whatever it's called where statistics are treated (compression of text is also rather important in signal processing).
The first one, V = k * n ^ b, is akin to the area of a circle, A = pi * r ^ 2, but inverted (taking the square root) and with a random factor, instead of pi, which can be pictured various ways, e.g. as a circle projected onto a wavy area or through a lense (doesn't really matter unless there's a specific need). If b is not exactly 0.5 the picture is a little different, but not really. The point is, this appears as the inverse square law, e.g. if a light cone hits a wall further away, the radius will increase linearly, but the power per square are will diminish proportionally with the inverse square of the distance. A^1/2 ~ r. The length of a text, n, increases likewise proportionally with the number of new words, n^0.5 ~ V. In other words, the text grows squarely with each new new word. That's also proportional to the circumference.
The second one seems more elaborate. I too have no idea what the extra variables are. Removing the logarithm we have *f(w) = C * (r(w)-b)^(-alpha)*. And transposed 1/C * (r(w)-b)^a = 1 / f(w). This is in principle the same polynomial form as V=K*n^b with several new parameters, if it were that V = 1 / f(w), k = 1/C, n = (r(w) - b), beta = -alpha.
There are a few notable differences. What's with those parameters? I'd assume the following:
b is likely a threshold under which the distribution is useless, because if r(w)Basic vocabulary.
If C is a constant as usual, then writing log(C), which would be constant as well, might just be a courtesy to ease solving for (w). It's inversely proportional to k, but that shouldn't trouble us now. One way or another it will be normalizing the results. I'm keen to assume that it means Corpus, but that gives me troubles. [todo]
That leaves alpha to be explained, which seems to be a variable nudge factor determined per corpus by a specific statistical procedure for error correction.
The last one is crucial. Raising to a negative power of alpha (=reciproke of the power of alpha) is not quite the same as taking the square root (power of 0.5). But it is similar in effect because the ranges of the exponents are also different, b < 1 < alpha. The very important difference is that the number of new words will tend to zero as the number of typed words tends to infinity--which, if I may say so, is a rather real possibility with twitter ;-) While the old formula would require ever new words to grow the text.
Somehow I'm trying to see 1/f as a derivative, compared to mechanical accelleration. But I'll rather leave the rest of the exercise to the reader. Please add a link to the video to your question. thx bye
add a comment |
I am going to guess and I hope someone has a clearer idea.
The question is interesting from a (my) novice math perspective, the wording suggests it was moved from mathoverflow.se?
From a basic linguistic perspective, there is little to no difference, all you need is a slowly decreasing slope. Both describe standard distributions, a concepts that's naturally observed in nature, from the distribution of raindrops to the dispersion of a laser beam. The specific choice depends on an accurate model. If it's instead just chosen to fit the data somehow, it doesn't hold much explanatory power, but it's a heuristic. For the specifics you should check out datascience.se, or whatever it's called where statistics are treated (compression of text is also rather important in signal processing).
The first one, V = k * n ^ b, is akin to the area of a circle, A = pi * r ^ 2, but inverted (taking the square root) and with a random factor, instead of pi, which can be pictured various ways, e.g. as a circle projected onto a wavy area or through a lense (doesn't really matter unless there's a specific need). If b is not exactly 0.5 the picture is a little different, but not really. The point is, this appears as the inverse square law, e.g. if a light cone hits a wall further away, the radius will increase linearly, but the power per square are will diminish proportionally with the inverse square of the distance. A^1/2 ~ r. The length of a text, n, increases likewise proportionally with the number of new words, n^0.5 ~ V. In other words, the text grows squarely with each new new word. That's also proportional to the circumference.
The second one seems more elaborate. I too have no idea what the extra variables are. Removing the logarithm we have *f(w) = C * (r(w)-b)^(-alpha)*. And transposed 1/C * (r(w)-b)^a = 1 / f(w). This is in principle the same polynomial form as V=K*n^b with several new parameters, if it were that V = 1 / f(w), k = 1/C, n = (r(w) - b), beta = -alpha.
There are a few notable differences. What's with those parameters? I'd assume the following:
b is likely a threshold under which the distribution is useless, because if r(w)Basic vocabulary.
If C is a constant as usual, then writing log(C), which would be constant as well, might just be a courtesy to ease solving for (w). It's inversely proportional to k, but that shouldn't trouble us now. One way or another it will be normalizing the results. I'm keen to assume that it means Corpus, but that gives me troubles. [todo]
That leaves alpha to be explained, which seems to be a variable nudge factor determined per corpus by a specific statistical procedure for error correction.
The last one is crucial. Raising to a negative power of alpha (=reciproke of the power of alpha) is not quite the same as taking the square root (power of 0.5). But it is similar in effect because the ranges of the exponents are also different, b < 1 < alpha. The very important difference is that the number of new words will tend to zero as the number of typed words tends to infinity--which, if I may say so, is a rather real possibility with twitter ;-) While the old formula would require ever new words to grow the text.
Somehow I'm trying to see 1/f as a derivative, compared to mechanical accelleration. But I'll rather leave the rest of the exercise to the reader. Please add a link to the video to your question. thx bye
add a comment |
I am going to guess and I hope someone has a clearer idea.
The question is interesting from a (my) novice math perspective, the wording suggests it was moved from mathoverflow.se?
From a basic linguistic perspective, there is little to no difference, all you need is a slowly decreasing slope. Both describe standard distributions, a concepts that's naturally observed in nature, from the distribution of raindrops to the dispersion of a laser beam. The specific choice depends on an accurate model. If it's instead just chosen to fit the data somehow, it doesn't hold much explanatory power, but it's a heuristic. For the specifics you should check out datascience.se, or whatever it's called where statistics are treated (compression of text is also rather important in signal processing).
The first one, V = k * n ^ b, is akin to the area of a circle, A = pi * r ^ 2, but inverted (taking the square root) and with a random factor, instead of pi, which can be pictured various ways, e.g. as a circle projected onto a wavy area or through a lense (doesn't really matter unless there's a specific need). If b is not exactly 0.5 the picture is a little different, but not really. The point is, this appears as the inverse square law, e.g. if a light cone hits a wall further away, the radius will increase linearly, but the power per square are will diminish proportionally with the inverse square of the distance. A^1/2 ~ r. The length of a text, n, increases likewise proportionally with the number of new words, n^0.5 ~ V. In other words, the text grows squarely with each new new word. That's also proportional to the circumference.
The second one seems more elaborate. I too have no idea what the extra variables are. Removing the logarithm we have *f(w) = C * (r(w)-b)^(-alpha)*. And transposed 1/C * (r(w)-b)^a = 1 / f(w). This is in principle the same polynomial form as V=K*n^b with several new parameters, if it were that V = 1 / f(w), k = 1/C, n = (r(w) - b), beta = -alpha.
There are a few notable differences. What's with those parameters? I'd assume the following:
b is likely a threshold under which the distribution is useless, because if r(w)Basic vocabulary.
If C is a constant as usual, then writing log(C), which would be constant as well, might just be a courtesy to ease solving for (w). It's inversely proportional to k, but that shouldn't trouble us now. One way or another it will be normalizing the results. I'm keen to assume that it means Corpus, but that gives me troubles. [todo]
That leaves alpha to be explained, which seems to be a variable nudge factor determined per corpus by a specific statistical procedure for error correction.
The last one is crucial. Raising to a negative power of alpha (=reciproke of the power of alpha) is not quite the same as taking the square root (power of 0.5). But it is similar in effect because the ranges of the exponents are also different, b < 1 < alpha. The very important difference is that the number of new words will tend to zero as the number of typed words tends to infinity--which, if I may say so, is a rather real possibility with twitter ;-) While the old formula would require ever new words to grow the text.
Somehow I'm trying to see 1/f as a derivative, compared to mechanical accelleration. But I'll rather leave the rest of the exercise to the reader. Please add a link to the video to your question. thx bye
I am going to guess and I hope someone has a clearer idea.
The question is interesting from a (my) novice math perspective, the wording suggests it was moved from mathoverflow.se?
From a basic linguistic perspective, there is little to no difference, all you need is a slowly decreasing slope. Both describe standard distributions, a concepts that's naturally observed in nature, from the distribution of raindrops to the dispersion of a laser beam. The specific choice depends on an accurate model. If it's instead just chosen to fit the data somehow, it doesn't hold much explanatory power, but it's a heuristic. For the specifics you should check out datascience.se, or whatever it's called where statistics are treated (compression of text is also rather important in signal processing).
The first one, V = k * n ^ b, is akin to the area of a circle, A = pi * r ^ 2, but inverted (taking the square root) and with a random factor, instead of pi, which can be pictured various ways, e.g. as a circle projected onto a wavy area or through a lense (doesn't really matter unless there's a specific need). If b is not exactly 0.5 the picture is a little different, but not really. The point is, this appears as the inverse square law, e.g. if a light cone hits a wall further away, the radius will increase linearly, but the power per square are will diminish proportionally with the inverse square of the distance. A^1/2 ~ r. The length of a text, n, increases likewise proportionally with the number of new words, n^0.5 ~ V. In other words, the text grows squarely with each new new word. That's also proportional to the circumference.
The second one seems more elaborate. I too have no idea what the extra variables are. Removing the logarithm we have *f(w) = C * (r(w)-b)^(-alpha)*. And transposed 1/C * (r(w)-b)^a = 1 / f(w). This is in principle the same polynomial form as V=K*n^b with several new parameters, if it were that V = 1 / f(w), k = 1/C, n = (r(w) - b), beta = -alpha.
There are a few notable differences. What's with those parameters? I'd assume the following:
b is likely a threshold under which the distribution is useless, because if r(w)Basic vocabulary.
If C is a constant as usual, then writing log(C), which would be constant as well, might just be a courtesy to ease solving for (w). It's inversely proportional to k, but that shouldn't trouble us now. One way or another it will be normalizing the results. I'm keen to assume that it means Corpus, but that gives me troubles. [todo]
That leaves alpha to be explained, which seems to be a variable nudge factor determined per corpus by a specific statistical procedure for error correction.
The last one is crucial. Raising to a negative power of alpha (=reciproke of the power of alpha) is not quite the same as taking the square root (power of 0.5). But it is similar in effect because the ranges of the exponents are also different, b < 1 < alpha. The very important difference is that the number of new words will tend to zero as the number of typed words tends to infinity--which, if I may say so, is a rather real possibility with twitter ;-) While the old formula would require ever new words to grow the text.
Somehow I'm trying to see 1/f as a derivative, compared to mechanical accelleration. But I'll rather leave the rest of the exercise to the reader. Please add a link to the video to your question. thx bye
answered Apr 9 at 17:48


vectoryvectory
40212
40212
add a comment |
add a comment |
Thanks for contributing an answer to Linguistics Stack Exchange!
- Please be sure to answer the question. Provide details and share your research!
But avoid …
- Asking for help, clarification, or responding to other answers.
- Making statements based on opinion; back them up with references or personal experience.
To learn more, see our tips on writing great answers.
Sign up or log in
StackExchange.ready(function ()
StackExchange.helpers.onClickDraftSave('#login-link');
);
Sign up using Google
Sign up using Facebook
Sign up using Email and Password
Post as a guest
Required, but never shown
StackExchange.ready(
function ()
StackExchange.openid.initPostLogin('.new-post-login', 'https%3a%2f%2flinguistics.stackexchange.com%2fquestions%2f31118%2fhow-to-interpret-this-form-of-heaps-law%23new-answer', 'question_page');
);
Post as a guest
Required, but never shown
Sign up or log in
StackExchange.ready(function ()
StackExchange.helpers.onClickDraftSave('#login-link');
);
Sign up using Google
Sign up using Facebook
Sign up using Email and Password
Post as a guest
Required, but never shown
Sign up or log in
StackExchange.ready(function ()
StackExchange.helpers.onClickDraftSave('#login-link');
);
Sign up using Google
Sign up using Facebook
Sign up using Email and Password
Post as a guest
Required, but never shown
Sign up or log in
StackExchange.ready(function ()
StackExchange.helpers.onClickDraftSave('#login-link');
);
Sign up using Google
Sign up using Facebook
Sign up using Email and Password
Sign up using Google
Sign up using Facebook
Sign up using Email and Password
Post as a guest
Required, but never shown
Required, but never shown
Required, but never shown
Required, but never shown
Required, but never shown
Required, but never shown
Required, but never shown
Required, but never shown
Required, but never shown
u8 ATeYDhLq7W4kOdPVS3UJ7xOVUF tI xIic LN 3vieSQBG1IGlKS9