How does quantile regression compare to logistic regression with the variable split at the quantile? The 2019 Stack Overflow Developer Survey Results Are InAnalyzing Logistic Regression when not using a dichotomous dependent variableEstimating logistic regression coefficients in a case-control design when the outcome variable is not case/control statusWhen does quantile regression produce biased coefficients (if ever)?How can I account for a nonlinear variable in a logistic regression?Variable Selection for Logistic regressionlogistic regression: the relation between sample proportion and prediction?How to compare the performance of two classification methods? (logistic regression and classification trees)Unbalanced Design with a Large Data Set and Logistic RegressionFit logistic regression with linear constraints on coefficients in RLogistic regression with double censored independent variable
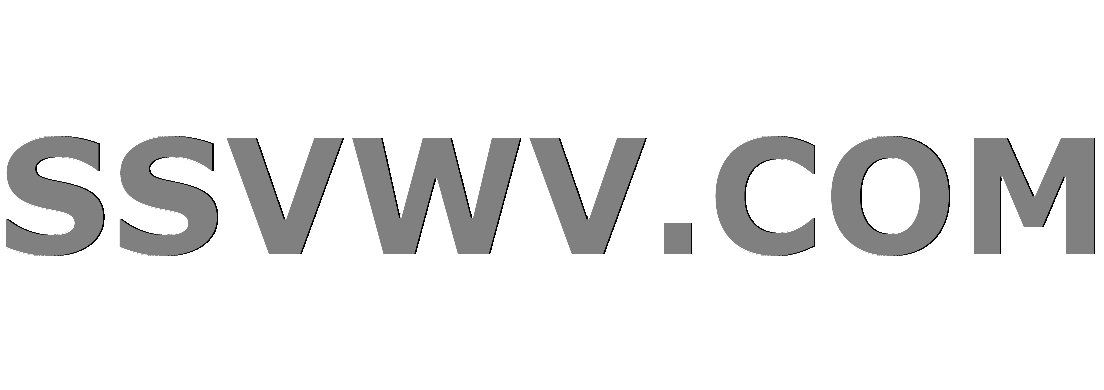
Multi tool use
Is it possible for absolutely everyone to attain enlightenment?
Can a rogue use sneak attack with weapons that have the thrown property even if they are not thrown?
Can we generate random numbers using irrational numbers like π and e?
Geography at the pixel level
Is it safe to harvest rainwater that fell on solar panels?
Cooking pasta in a water boiler
How safe is it to go to Baghdad?
I am an eight letter word. What am I?
Is it ok to offer lower paid work as a trial period before negotiating for a full-time job?
What is the meaning of Triage in Cybersec world?
How to notate time signature switching consistently every measure
Can withdrawing asylum be illegal?
What is the most efficient way to store a numeric range?
What is this sharp, curved notch on my knife for?
Why doesn't UInt have a toDouble()?
Why doesn't mkfifo with a mode of 1755 grant read permissions and sticky bit to the user?
Why was M87 targeted for the Event Horizon Telescope instead of Sagittarius A*?
Can a flute soloist sit?
Worn-tile Scrabble
Are spiders unable to hurt humans, especially very small spiders?
Straighten subgroup lattice
What could be the right powersource for 15 seconds lifespan disposable giant chainsaw?
Button changing its text & action. Good or terrible?
Sums of normal random variables
How does quantile regression compare to logistic regression with the variable split at the quantile?
The 2019 Stack Overflow Developer Survey Results Are InAnalyzing Logistic Regression when not using a dichotomous dependent variableEstimating logistic regression coefficients in a case-control design when the outcome variable is not case/control statusWhen does quantile regression produce biased coefficients (if ever)?How can I account for a nonlinear variable in a logistic regression?Variable Selection for Logistic regressionlogistic regression: the relation between sample proportion and prediction?How to compare the performance of two classification methods? (logistic regression and classification trees)Unbalanced Design with a Large Data Set and Logistic RegressionFit logistic regression with linear constraints on coefficients in RLogistic regression with double censored independent variable
.everyoneloves__top-leaderboard:empty,.everyoneloves__mid-leaderboard:empty,.everyoneloves__bot-mid-leaderboard:empty margin-bottom:0;
$begingroup$
I googled a bit but didn't find anything on this.
Suppose you do a quantile regression on the qth quantile of the dependent variable.
Then you split the DV at the qth quantile and label the result 0 and 1. Then you do logistic regression on the categorized DV.
I'm looking for any Monte-Carlo studies of this or reasons to prefer one over the other etc.
logistic quantile-regression
$endgroup$
add a comment |
$begingroup$
I googled a bit but didn't find anything on this.
Suppose you do a quantile regression on the qth quantile of the dependent variable.
Then you split the DV at the qth quantile and label the result 0 and 1. Then you do logistic regression on the categorized DV.
I'm looking for any Monte-Carlo studies of this or reasons to prefer one over the other etc.
logistic quantile-regression
$endgroup$
2
$begingroup$
Could you show us any reasonable way even to compare the results of the two regressions? After all, unless you have something a little less general in mind, the coefficients of the regressors in these two models have entirely different meanings and interpretations, so in what sense are we to understand what you mean by "prefer"?
$endgroup$
– whuber♦
Apr 5 at 21:48
add a comment |
$begingroup$
I googled a bit but didn't find anything on this.
Suppose you do a quantile regression on the qth quantile of the dependent variable.
Then you split the DV at the qth quantile and label the result 0 and 1. Then you do logistic regression on the categorized DV.
I'm looking for any Monte-Carlo studies of this or reasons to prefer one over the other etc.
logistic quantile-regression
$endgroup$
I googled a bit but didn't find anything on this.
Suppose you do a quantile regression on the qth quantile of the dependent variable.
Then you split the DV at the qth quantile and label the result 0 and 1. Then you do logistic regression on the categorized DV.
I'm looking for any Monte-Carlo studies of this or reasons to prefer one over the other etc.
logistic quantile-regression
logistic quantile-regression
asked Apr 5 at 19:50
Peter Flom♦Peter Flom
77.3k12109217
77.3k12109217
2
$begingroup$
Could you show us any reasonable way even to compare the results of the two regressions? After all, unless you have something a little less general in mind, the coefficients of the regressors in these two models have entirely different meanings and interpretations, so in what sense are we to understand what you mean by "prefer"?
$endgroup$
– whuber♦
Apr 5 at 21:48
add a comment |
2
$begingroup$
Could you show us any reasonable way even to compare the results of the two regressions? After all, unless you have something a little less general in mind, the coefficients of the regressors in these two models have entirely different meanings and interpretations, so in what sense are we to understand what you mean by "prefer"?
$endgroup$
– whuber♦
Apr 5 at 21:48
2
2
$begingroup$
Could you show us any reasonable way even to compare the results of the two regressions? After all, unless you have something a little less general in mind, the coefficients of the regressors in these two models have entirely different meanings and interpretations, so in what sense are we to understand what you mean by "prefer"?
$endgroup$
– whuber♦
Apr 5 at 21:48
$begingroup$
Could you show us any reasonable way even to compare the results of the two regressions? After all, unless you have something a little less general in mind, the coefficients of the regressors in these two models have entirely different meanings and interpretations, so in what sense are we to understand what you mean by "prefer"?
$endgroup$
– whuber♦
Apr 5 at 21:48
add a comment |
3 Answers
3
active
oldest
votes
$begingroup$
For simplicity, assume you have a continuous dependent variable Y and a continuous predictor variable X.
Logistic Regression
If I understand your post correctly, your logistic regression will categorize Y into 0 and 1 based on the quantile of the (unconditional) distribution of Y. Specifically, the q-th quantile of the distribution of observed Y values will be computed and Ycat will be defined as 0 if Y is strictly less than this quantile and 1 if Y is greater than or equal to this quantile.
If the above captures your intent, then the logistic regression will model the odds of Y exceeding or being equal to the (observed) q-th quantile of the (unconditional) Y distribution as a function of X.
Quantile Regression
On the other hand, if you are performing a quantile regression of Y on X, you are focusing on modelling how the q-th quantile of the conditional distribution of Y given X changes as a function of X.
Logistic Regression versus Quantile Regression
It seems to me that these two procedures have totally different aims, since the first procedure (i.e., logistic regression) focuses on the q-th quantile of the unconditional distribution of Y, whereas the second procedure (i.e., quantile regression) focuses on the the q-th quantile of the conditional distribution of Y.
The unconditional distribution of Y is the
distribution of Y values (hence it ignores any
information about the X values).
The conditional distribution of Y given X is the
distribution of those Y values for which the values
of X are the same.
Illustrative Example
For illustration purposes, let's say Y = cholesterol and X = body weight.
Then logistic regression is modelling the odds of having a 'high' cholesterol value (i.e., greater than or equal to the q-th quantile of the observed cholesterol values) as a function of body weight, where the definition of 'high' has no relation to body weight. In other words, the marker for what constitutes a 'high' cholesterol value is independent of body weight. What changes with body weight in this model is the odds that a cholesterol value would exceed this marker.
On the other hand, quantile regression is looking at how the 'marker' cholesterol values for which q% of the subjects with the same body weight in the underlying population have a higher cholesterol value vary as a function of body weight. You can think of these cholesterol values as markers for identifying what cholesterol values are 'high' - but in this case, each marker depends on the corresponding body weight; furthermore, the markers are assumed to change in a predictable fashion as the value of X changes (e.g., the markers tend to increase as X increases).
$endgroup$
2
$begingroup$
I agree with all that. Yet, there does seem to be a similarity - that is, both look at the qth quantile as a function of the same independent variables.
$endgroup$
– Peter Flom♦
Apr 5 at 22:16
4
$begingroup$
Yes, but the difference is that one method looks at the unconditional quantile (i.e., logistic regression) while the other looks at the conditional quantile (i.e., quantile regression). Those two quantiles keep track of different things.
$endgroup$
– Isabella Ghement
Apr 5 at 22:19
add a comment |
$begingroup$
They won't be equal, and the reason is simple.
With quantile regression you want to model the quantile conditional of the independent variables. Your approach with logistic regression fits the marginal quantile.
$endgroup$
add a comment |
$begingroup$
One asks "what is the effect on the nth quantile of the dependent variable's distribution?" The other one asks "what is the effect on the probability that the dependent variable falls into the nth quantile of its unconditional distribution?"
I.e., the fact that they both have the word "quantile" in them let's them look more similar than they are.
I guess if you first estimate a conditional quantile function, use this for the split and proceed from there, the two approaches would become more similar. But I don't see what you would stand to gain from such a detour.
.
$endgroup$
add a comment |
Your Answer
StackExchange.ifUsing("editor", function ()
return StackExchange.using("mathjaxEditing", function ()
StackExchange.MarkdownEditor.creationCallbacks.add(function (editor, postfix)
StackExchange.mathjaxEditing.prepareWmdForMathJax(editor, postfix, [["$", "$"], ["\\(","\\)"]]);
);
);
, "mathjax-editing");
StackExchange.ready(function()
var channelOptions =
tags: "".split(" "),
id: "65"
;
initTagRenderer("".split(" "), "".split(" "), channelOptions);
StackExchange.using("externalEditor", function()
// Have to fire editor after snippets, if snippets enabled
if (StackExchange.settings.snippets.snippetsEnabled)
StackExchange.using("snippets", function()
createEditor();
);
else
createEditor();
);
function createEditor()
StackExchange.prepareEditor(
heartbeatType: 'answer',
autoActivateHeartbeat: false,
convertImagesToLinks: false,
noModals: true,
showLowRepImageUploadWarning: true,
reputationToPostImages: null,
bindNavPrevention: true,
postfix: "",
imageUploader:
brandingHtml: "Powered by u003ca class="icon-imgur-white" href="https://imgur.com/"u003eu003c/au003e",
contentPolicyHtml: "User contributions licensed under u003ca href="https://creativecommons.org/licenses/by-sa/3.0/"u003ecc by-sa 3.0 with attribution requiredu003c/au003e u003ca href="https://stackoverflow.com/legal/content-policy"u003e(content policy)u003c/au003e",
allowUrls: true
,
onDemand: true,
discardSelector: ".discard-answer"
,immediatelyShowMarkdownHelp:true
);
);
Sign up or log in
StackExchange.ready(function ()
StackExchange.helpers.onClickDraftSave('#login-link');
);
Sign up using Google
Sign up using Facebook
Sign up using Email and Password
Post as a guest
Required, but never shown
StackExchange.ready(
function ()
StackExchange.openid.initPostLogin('.new-post-login', 'https%3a%2f%2fstats.stackexchange.com%2fquestions%2f401421%2fhow-does-quantile-regression-compare-to-logistic-regression-with-the-variable-sp%23new-answer', 'question_page');
);
Post as a guest
Required, but never shown
3 Answers
3
active
oldest
votes
3 Answers
3
active
oldest
votes
active
oldest
votes
active
oldest
votes
$begingroup$
For simplicity, assume you have a continuous dependent variable Y and a continuous predictor variable X.
Logistic Regression
If I understand your post correctly, your logistic regression will categorize Y into 0 and 1 based on the quantile of the (unconditional) distribution of Y. Specifically, the q-th quantile of the distribution of observed Y values will be computed and Ycat will be defined as 0 if Y is strictly less than this quantile and 1 if Y is greater than or equal to this quantile.
If the above captures your intent, then the logistic regression will model the odds of Y exceeding or being equal to the (observed) q-th quantile of the (unconditional) Y distribution as a function of X.
Quantile Regression
On the other hand, if you are performing a quantile regression of Y on X, you are focusing on modelling how the q-th quantile of the conditional distribution of Y given X changes as a function of X.
Logistic Regression versus Quantile Regression
It seems to me that these two procedures have totally different aims, since the first procedure (i.e., logistic regression) focuses on the q-th quantile of the unconditional distribution of Y, whereas the second procedure (i.e., quantile regression) focuses on the the q-th quantile of the conditional distribution of Y.
The unconditional distribution of Y is the
distribution of Y values (hence it ignores any
information about the X values).
The conditional distribution of Y given X is the
distribution of those Y values for which the values
of X are the same.
Illustrative Example
For illustration purposes, let's say Y = cholesterol and X = body weight.
Then logistic regression is modelling the odds of having a 'high' cholesterol value (i.e., greater than or equal to the q-th quantile of the observed cholesterol values) as a function of body weight, where the definition of 'high' has no relation to body weight. In other words, the marker for what constitutes a 'high' cholesterol value is independent of body weight. What changes with body weight in this model is the odds that a cholesterol value would exceed this marker.
On the other hand, quantile regression is looking at how the 'marker' cholesterol values for which q% of the subjects with the same body weight in the underlying population have a higher cholesterol value vary as a function of body weight. You can think of these cholesterol values as markers for identifying what cholesterol values are 'high' - but in this case, each marker depends on the corresponding body weight; furthermore, the markers are assumed to change in a predictable fashion as the value of X changes (e.g., the markers tend to increase as X increases).
$endgroup$
2
$begingroup$
I agree with all that. Yet, there does seem to be a similarity - that is, both look at the qth quantile as a function of the same independent variables.
$endgroup$
– Peter Flom♦
Apr 5 at 22:16
4
$begingroup$
Yes, but the difference is that one method looks at the unconditional quantile (i.e., logistic regression) while the other looks at the conditional quantile (i.e., quantile regression). Those two quantiles keep track of different things.
$endgroup$
– Isabella Ghement
Apr 5 at 22:19
add a comment |
$begingroup$
For simplicity, assume you have a continuous dependent variable Y and a continuous predictor variable X.
Logistic Regression
If I understand your post correctly, your logistic regression will categorize Y into 0 and 1 based on the quantile of the (unconditional) distribution of Y. Specifically, the q-th quantile of the distribution of observed Y values will be computed and Ycat will be defined as 0 if Y is strictly less than this quantile and 1 if Y is greater than or equal to this quantile.
If the above captures your intent, then the logistic regression will model the odds of Y exceeding or being equal to the (observed) q-th quantile of the (unconditional) Y distribution as a function of X.
Quantile Regression
On the other hand, if you are performing a quantile regression of Y on X, you are focusing on modelling how the q-th quantile of the conditional distribution of Y given X changes as a function of X.
Logistic Regression versus Quantile Regression
It seems to me that these two procedures have totally different aims, since the first procedure (i.e., logistic regression) focuses on the q-th quantile of the unconditional distribution of Y, whereas the second procedure (i.e., quantile regression) focuses on the the q-th quantile of the conditional distribution of Y.
The unconditional distribution of Y is the
distribution of Y values (hence it ignores any
information about the X values).
The conditional distribution of Y given X is the
distribution of those Y values for which the values
of X are the same.
Illustrative Example
For illustration purposes, let's say Y = cholesterol and X = body weight.
Then logistic regression is modelling the odds of having a 'high' cholesterol value (i.e., greater than or equal to the q-th quantile of the observed cholesterol values) as a function of body weight, where the definition of 'high' has no relation to body weight. In other words, the marker for what constitutes a 'high' cholesterol value is independent of body weight. What changes with body weight in this model is the odds that a cholesterol value would exceed this marker.
On the other hand, quantile regression is looking at how the 'marker' cholesterol values for which q% of the subjects with the same body weight in the underlying population have a higher cholesterol value vary as a function of body weight. You can think of these cholesterol values as markers for identifying what cholesterol values are 'high' - but in this case, each marker depends on the corresponding body weight; furthermore, the markers are assumed to change in a predictable fashion as the value of X changes (e.g., the markers tend to increase as X increases).
$endgroup$
2
$begingroup$
I agree with all that. Yet, there does seem to be a similarity - that is, both look at the qth quantile as a function of the same independent variables.
$endgroup$
– Peter Flom♦
Apr 5 at 22:16
4
$begingroup$
Yes, but the difference is that one method looks at the unconditional quantile (i.e., logistic regression) while the other looks at the conditional quantile (i.e., quantile regression). Those two quantiles keep track of different things.
$endgroup$
– Isabella Ghement
Apr 5 at 22:19
add a comment |
$begingroup$
For simplicity, assume you have a continuous dependent variable Y and a continuous predictor variable X.
Logistic Regression
If I understand your post correctly, your logistic regression will categorize Y into 0 and 1 based on the quantile of the (unconditional) distribution of Y. Specifically, the q-th quantile of the distribution of observed Y values will be computed and Ycat will be defined as 0 if Y is strictly less than this quantile and 1 if Y is greater than or equal to this quantile.
If the above captures your intent, then the logistic regression will model the odds of Y exceeding or being equal to the (observed) q-th quantile of the (unconditional) Y distribution as a function of X.
Quantile Regression
On the other hand, if you are performing a quantile regression of Y on X, you are focusing on modelling how the q-th quantile of the conditional distribution of Y given X changes as a function of X.
Logistic Regression versus Quantile Regression
It seems to me that these two procedures have totally different aims, since the first procedure (i.e., logistic regression) focuses on the q-th quantile of the unconditional distribution of Y, whereas the second procedure (i.e., quantile regression) focuses on the the q-th quantile of the conditional distribution of Y.
The unconditional distribution of Y is the
distribution of Y values (hence it ignores any
information about the X values).
The conditional distribution of Y given X is the
distribution of those Y values for which the values
of X are the same.
Illustrative Example
For illustration purposes, let's say Y = cholesterol and X = body weight.
Then logistic regression is modelling the odds of having a 'high' cholesterol value (i.e., greater than or equal to the q-th quantile of the observed cholesterol values) as a function of body weight, where the definition of 'high' has no relation to body weight. In other words, the marker for what constitutes a 'high' cholesterol value is independent of body weight. What changes with body weight in this model is the odds that a cholesterol value would exceed this marker.
On the other hand, quantile regression is looking at how the 'marker' cholesterol values for which q% of the subjects with the same body weight in the underlying population have a higher cholesterol value vary as a function of body weight. You can think of these cholesterol values as markers for identifying what cholesterol values are 'high' - but in this case, each marker depends on the corresponding body weight; furthermore, the markers are assumed to change in a predictable fashion as the value of X changes (e.g., the markers tend to increase as X increases).
$endgroup$
For simplicity, assume you have a continuous dependent variable Y and a continuous predictor variable X.
Logistic Regression
If I understand your post correctly, your logistic regression will categorize Y into 0 and 1 based on the quantile of the (unconditional) distribution of Y. Specifically, the q-th quantile of the distribution of observed Y values will be computed and Ycat will be defined as 0 if Y is strictly less than this quantile and 1 if Y is greater than or equal to this quantile.
If the above captures your intent, then the logistic regression will model the odds of Y exceeding or being equal to the (observed) q-th quantile of the (unconditional) Y distribution as a function of X.
Quantile Regression
On the other hand, if you are performing a quantile regression of Y on X, you are focusing on modelling how the q-th quantile of the conditional distribution of Y given X changes as a function of X.
Logistic Regression versus Quantile Regression
It seems to me that these two procedures have totally different aims, since the first procedure (i.e., logistic regression) focuses on the q-th quantile of the unconditional distribution of Y, whereas the second procedure (i.e., quantile regression) focuses on the the q-th quantile of the conditional distribution of Y.
The unconditional distribution of Y is the
distribution of Y values (hence it ignores any
information about the X values).
The conditional distribution of Y given X is the
distribution of those Y values for which the values
of X are the same.
Illustrative Example
For illustration purposes, let's say Y = cholesterol and X = body weight.
Then logistic regression is modelling the odds of having a 'high' cholesterol value (i.e., greater than or equal to the q-th quantile of the observed cholesterol values) as a function of body weight, where the definition of 'high' has no relation to body weight. In other words, the marker for what constitutes a 'high' cholesterol value is independent of body weight. What changes with body weight in this model is the odds that a cholesterol value would exceed this marker.
On the other hand, quantile regression is looking at how the 'marker' cholesterol values for which q% of the subjects with the same body weight in the underlying population have a higher cholesterol value vary as a function of body weight. You can think of these cholesterol values as markers for identifying what cholesterol values are 'high' - but in this case, each marker depends on the corresponding body weight; furthermore, the markers are assumed to change in a predictable fashion as the value of X changes (e.g., the markers tend to increase as X increases).
edited Apr 8 at 9:33
Richard Hardy
28.2k642129
28.2k642129
answered Apr 5 at 20:29


Isabella GhementIsabella Ghement
7,9661422
7,9661422
2
$begingroup$
I agree with all that. Yet, there does seem to be a similarity - that is, both look at the qth quantile as a function of the same independent variables.
$endgroup$
– Peter Flom♦
Apr 5 at 22:16
4
$begingroup$
Yes, but the difference is that one method looks at the unconditional quantile (i.e., logistic regression) while the other looks at the conditional quantile (i.e., quantile regression). Those two quantiles keep track of different things.
$endgroup$
– Isabella Ghement
Apr 5 at 22:19
add a comment |
2
$begingroup$
I agree with all that. Yet, there does seem to be a similarity - that is, both look at the qth quantile as a function of the same independent variables.
$endgroup$
– Peter Flom♦
Apr 5 at 22:16
4
$begingroup$
Yes, but the difference is that one method looks at the unconditional quantile (i.e., logistic regression) while the other looks at the conditional quantile (i.e., quantile regression). Those two quantiles keep track of different things.
$endgroup$
– Isabella Ghement
Apr 5 at 22:19
2
2
$begingroup$
I agree with all that. Yet, there does seem to be a similarity - that is, both look at the qth quantile as a function of the same independent variables.
$endgroup$
– Peter Flom♦
Apr 5 at 22:16
$begingroup$
I agree with all that. Yet, there does seem to be a similarity - that is, both look at the qth quantile as a function of the same independent variables.
$endgroup$
– Peter Flom♦
Apr 5 at 22:16
4
4
$begingroup$
Yes, but the difference is that one method looks at the unconditional quantile (i.e., logistic regression) while the other looks at the conditional quantile (i.e., quantile regression). Those two quantiles keep track of different things.
$endgroup$
– Isabella Ghement
Apr 5 at 22:19
$begingroup$
Yes, but the difference is that one method looks at the unconditional quantile (i.e., logistic regression) while the other looks at the conditional quantile (i.e., quantile regression). Those two quantiles keep track of different things.
$endgroup$
– Isabella Ghement
Apr 5 at 22:19
add a comment |
$begingroup$
They won't be equal, and the reason is simple.
With quantile regression you want to model the quantile conditional of the independent variables. Your approach with logistic regression fits the marginal quantile.
$endgroup$
add a comment |
$begingroup$
They won't be equal, and the reason is simple.
With quantile regression you want to model the quantile conditional of the independent variables. Your approach with logistic regression fits the marginal quantile.
$endgroup$
add a comment |
$begingroup$
They won't be equal, and the reason is simple.
With quantile regression you want to model the quantile conditional of the independent variables. Your approach with logistic regression fits the marginal quantile.
$endgroup$
They won't be equal, and the reason is simple.
With quantile regression you want to model the quantile conditional of the independent variables. Your approach with logistic regression fits the marginal quantile.
answered Apr 5 at 22:54


FirebugFirebug
7,76123280
7,76123280
add a comment |
add a comment |
$begingroup$
One asks "what is the effect on the nth quantile of the dependent variable's distribution?" The other one asks "what is the effect on the probability that the dependent variable falls into the nth quantile of its unconditional distribution?"
I.e., the fact that they both have the word "quantile" in them let's them look more similar than they are.
I guess if you first estimate a conditional quantile function, use this for the split and proceed from there, the two approaches would become more similar. But I don't see what you would stand to gain from such a detour.
.
$endgroup$
add a comment |
$begingroup$
One asks "what is the effect on the nth quantile of the dependent variable's distribution?" The other one asks "what is the effect on the probability that the dependent variable falls into the nth quantile of its unconditional distribution?"
I.e., the fact that they both have the word "quantile" in them let's them look more similar than they are.
I guess if you first estimate a conditional quantile function, use this for the split and proceed from there, the two approaches would become more similar. But I don't see what you would stand to gain from such a detour.
.
$endgroup$
add a comment |
$begingroup$
One asks "what is the effect on the nth quantile of the dependent variable's distribution?" The other one asks "what is the effect on the probability that the dependent variable falls into the nth quantile of its unconditional distribution?"
I.e., the fact that they both have the word "quantile" in them let's them look more similar than they are.
I guess if you first estimate a conditional quantile function, use this for the split and proceed from there, the two approaches would become more similar. But I don't see what you would stand to gain from such a detour.
.
$endgroup$
One asks "what is the effect on the nth quantile of the dependent variable's distribution?" The other one asks "what is the effect on the probability that the dependent variable falls into the nth quantile of its unconditional distribution?"
I.e., the fact that they both have the word "quantile" in them let's them look more similar than they are.
I guess if you first estimate a conditional quantile function, use this for the split and proceed from there, the two approaches would become more similar. But I don't see what you would stand to gain from such a detour.
.
answered Apr 6 at 8:06


sheßsheß
230419
230419
add a comment |
add a comment |
Thanks for contributing an answer to Cross Validated!
- Please be sure to answer the question. Provide details and share your research!
But avoid …
- Asking for help, clarification, or responding to other answers.
- Making statements based on opinion; back them up with references or personal experience.
Use MathJax to format equations. MathJax reference.
To learn more, see our tips on writing great answers.
Sign up or log in
StackExchange.ready(function ()
StackExchange.helpers.onClickDraftSave('#login-link');
);
Sign up using Google
Sign up using Facebook
Sign up using Email and Password
Post as a guest
Required, but never shown
StackExchange.ready(
function ()
StackExchange.openid.initPostLogin('.new-post-login', 'https%3a%2f%2fstats.stackexchange.com%2fquestions%2f401421%2fhow-does-quantile-regression-compare-to-logistic-regression-with-the-variable-sp%23new-answer', 'question_page');
);
Post as a guest
Required, but never shown
Sign up or log in
StackExchange.ready(function ()
StackExchange.helpers.onClickDraftSave('#login-link');
);
Sign up using Google
Sign up using Facebook
Sign up using Email and Password
Post as a guest
Required, but never shown
Sign up or log in
StackExchange.ready(function ()
StackExchange.helpers.onClickDraftSave('#login-link');
);
Sign up using Google
Sign up using Facebook
Sign up using Email and Password
Post as a guest
Required, but never shown
Sign up or log in
StackExchange.ready(function ()
StackExchange.helpers.onClickDraftSave('#login-link');
);
Sign up using Google
Sign up using Facebook
Sign up using Email and Password
Sign up using Google
Sign up using Facebook
Sign up using Email and Password
Post as a guest
Required, but never shown
Required, but never shown
Required, but never shown
Required, but never shown
Required, but never shown
Required, but never shown
Required, but never shown
Required, but never shown
Required, but never shown
txEqkKDJb3FWbLjN,K7a,Boz,UK7U g27L
2
$begingroup$
Could you show us any reasonable way even to compare the results of the two regressions? After all, unless you have something a little less general in mind, the coefficients of the regressors in these two models have entirely different meanings and interpretations, so in what sense are we to understand what you mean by "prefer"?
$endgroup$
– whuber♦
Apr 5 at 21:48